Correlation doesn’t equal causation. We all have this beaten into our heads by legions of Internet commenters who spam it under every science article.
But, why not?
Well, the simple answer is because just because A and B happen at around the same time doesn’t mean A causes B. B could cause A, or something else could cause both of them, or maybe they have nothing to do with each other.
If I was an Internet commentator, I’d just leave it there. But, let’s think like a scientist. What if we ruled out every possible other path from A to B, besides the path that A causes B? Would that mean that A causes B?
I mean, well, yeah, right? That’s kind of what a controlled experiment is for. It rules out every other possibility besides A causing B. If we, say, use a catapult to launch a boulder, we can say the movement of the catapult caused the boulder to be launched, even though technically the movement of the catapult was just correlated with the boulder. But we know for sure the boulder didn’t cause the movement of the catapult, and that the two didn’t just happen to both happen at around the same time.
This is an interesting, but not particularly useful way to think about an experiment. It can, however, be a very useful way to think about observations. If we observe A and B are correlated, and we rule out every other possibility other than A causing B, A has to have caused B.
This is exactly how Mendelian randomization works. Mendelian randomization is a way of showing that genetic variation can cause a certain outcome, like a certain “single nucleotide polymorphism” (a one letter substitution in the genetic code) can cause someone to be statistically more susceptible to heart attacks. We can use Mendelian randomization to show this by correlation alone, which is really neat. Let’s get into how we do it.
We start with a genetic variation. Let’s stick with our heart attack example, and say there’s a mutation in the ABCA1 gene1. We think that has something to do with heart attacks.
Our first task is to identify a way in which the ABCA1 gene could impact heart attacks. It’s obviously not going to impact heart attacks directly, but it could impact it through cholesterol levels, which we already know from prior experiments in mice that the ABCA1 gene impacts.
We now have our basic setup: we want to check if the gene variant’s impact on cholesterol levels impacts the chance of heart attacks. So, now we can take a bunch of people who have the genetic variant and who don’t, and measure their levels of cholesterol and their chance of heart attack. We’ll eventually get some statistics on who has the genetic variant, what their cholesterol levels are, and who got heart attacks.
Let’s say that, in our statistics, we find that people with a certain SNP have a statistically lower level of HDL cholesterol but an lower rate of heart attacks compared to normal people2. This is our correlation. Let’s run through our possible paths as to why this might be.
1) The SNP causes a decrease in cholesterol, which lowers the rate of heart attacks.
2) The SNP causes something else, which lowers the rate of heart attacks. The lowered cholesterol happens for some completely different reason.
3) The SNP directly lowers the rate of heart attacks. The lowered cholesterol happens for some completely different reason.
4) The SNP causes the decrease in cholesterol. Something else lowers the rate of heart attacks.
5) Something else causes the SNP, the lowered rate of cholesterol, and the heart attacks.
6) The SNP causes the decrease in cholesterol and also, through a separate mechanism, lowers the rate of heart attacks.
7) The lowered rate of cholesterol causes the SNP.
Our goal is to narrow it down to only 1. Let’s see what we can cross out.
Let’s start by crossing out 7. Genetic variations are fixed at birth, and definitely can’t be caused by something in the body.
Let’s also cross out 2, 3, and 5. We know from our experiments that this SNP directly impacts cholesterol.
This leaves us with 4 and 6 to get rid of. These are actually surprisingly difficult to deal with, as we need to make sure that it’s only the lowered cholesterol that impacts heart attacks and nothing else. The researcher needs to make sure their analysis controls for all other confounders, like by carefully matching up observations by subjects who are similar in every other way except for cholesterol. This does mean that we have to make a big assumption that we know every other way that heart attack rates can be impacted, which is not necessarily true.
But, if we can make that assumption3, we end with the only remaining path, which is the first option. This is the causative path: the SNP caused the decrease in cholesterol which lowered the rate of heart attacks. Or, to use Wikipedia’s handy diagram, we’re only left with Z caused X which caused Y:
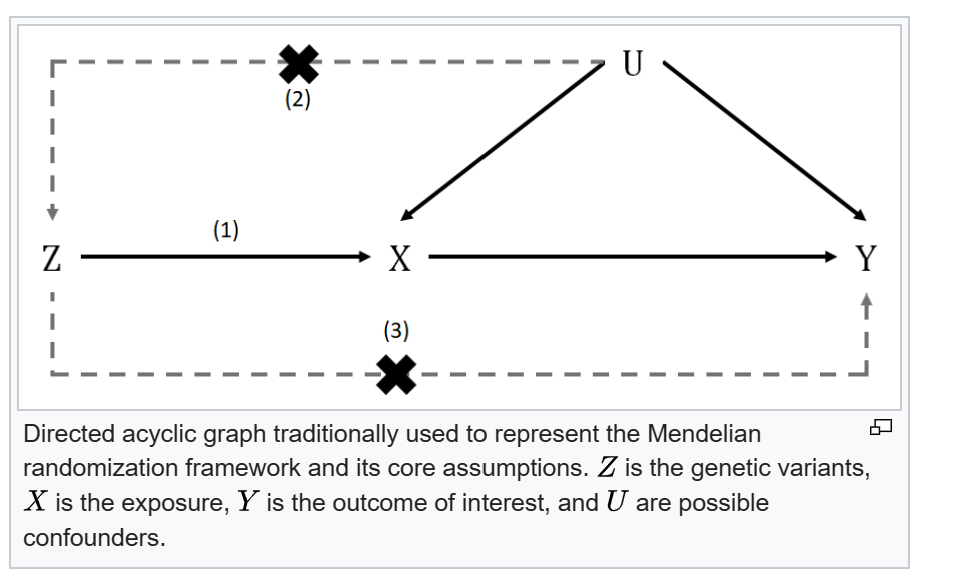
And that’s how we can prove causation from correlation in genetics, subject to a key assumption that we know all possible confounders. It’s pretty cool. Does it have any application outside of genetics?
I think so. As long as we can start with something that’s totally independently determined (e.g. we know that A causes B and B definitely does not cause A), and as long as we know there is a causal connection between A and B, we can apply this framework. I’ll be on the lookout for other places to apply it, and you can let me know if you have any ideas as well.
Taking this example from here.
In the real study linked in footnote 1, they found the opposite: the SNP lowers the rate of HDL cholesterol but does not impact the rate of heart attacks. This was actually an important way to prove that HDL cholesterol does not impact the rate of heart attacks, which added to the dietary debate about “good” and “bad” cholesterol. But this analysis is confusing enough without talking about proving a lack of causation.
Wikipedia lists 3 assumptions needed for Mendelizan randomization:
1. Relevance: the genetic variant causes the exposure. In our example, the relevance is that variation in the ABCA1 gene can cause levels of cholesterol to change as determined by mouse experiments.
2. Independence: nothing causes both the genetic variant and the outcome. That was a given for our example, and for almost any genetic condition.
3. No horizontal pleiotropy: the genetic variant doesn’t cause anything that would cause the outcome other than the exposure. That was the “big assumption” I mentioned, that the gene variant couldn’t affect heart attacks except through changes in cholesterol.